Overview
Welcome to The Burn Contributor's Book π
This book will help you get acquainted with the internals of the Burn deep learning framework and provide some detailed guidance on how to contribute to the project.
We have crafted some sections for you:
-
Getting Started: Much like the Burn Book which targets users, we'll start with the fundamentals, guiding you through tasks like setting up the development environment, running tests, and what you should check prior to each commit.
-
Project Architecture: This section will give you an in-depth look at the architecture of Burn.
-
Guides: We provide some guides on how to do specific tasks, such as adding a new operations to Burn.
-
Frequently Encountered Issues: If you are running into an issue that has you stumped, this is the section to check out prior to asking on the Discord. It's a collection of errors encountered by contributors, what caused them, and how they were resolved.
As this book is geared towards contributors and not towards users of Burn, we'll assume you have a good understanding of software development, but will make efforts to explain anything outside of that scope, or at least provide links to resources that explain it better than we can.
How to read this book
Throughout this book, we maintain the following structure.
Linking
When referring to structures or functions within codebase, we provide permalinks to the lines in
specific commits, and indicate them by the relative path of their parent file from the project root.
For example this is a reference to the Tensor
struct in
crates/burn-tensor/src/tensor/api/base.rs
When some reference information is useful but is beyond the scope of contributing to Burn, we
provide that information in a footnote. To build on the previous example, the Tensor
mentioned is
what's referred to as a newtype struct1.
Direct hyperlinks are for tools and resources that are not part of the Burn project, but are useful for contributing to it. For example, when working on implementing an operation for autodiff, it can be useful to use symbolab to calculate the left and right partial derivatives.
For more information on newtype please refer to the Advanced Types chapter of the Rust Book
Getting Started
This section is for setting up the environment and how to do basic development tasks such as running tests and checking your code before committing. If you need help with the process or run into issues, feel free to ask on the Discord server in the Development channels.
Setting up the environment
Depending on what part of the project you plan on contributing to, there are a couple of tools to install and commands to be familiar with. This section should be up to date with current project practices (as of 2024-04-15).
General
There are a few commands you will want to run prior to any commit for a non-draft PR:
cargo fmt --all
will runrustfmt
on all files in the project.cargo clippy --fix
will run Clippy and fix any coding issues it can. Clippy necessitates to be in a clean Git state, but this can be circumvented by adding the--allow-dirty
flag.cargo xtask check all
is a script located in the project root that builds and tests the project. It is required to run successfully prior to merging a PR. Fair warning, running these tests can take a while1.
Updating the burn semver version
If for some reason you need to bump for the next version (though that should probably be left to the
maintainers), edit the semantic version number in burn/Cargo.toml
, and then run cargo update
to
update the lock file.
Contributing to either the Burn Book or Contributor Book
Both the Burn Book and the Contributor Book are built with mdbook. To open the book locally, run
mdbook serve <path/to/book>
or cargo xtask books {burn|contributor} open
which will install and
use mdbook automatically.
Alternatively, if you want to install mdbook directly, run the following command2:
cargo install mdbook
Also instead of running cargo xtask check all
, you can run cargo xtask check typos
to
only check for misspellings. This will install typo, and if
any are encountered you should be able to run typo -w /path/to/book
to fix them.
If your system is running into issues with memory and you are on linux, you may want to switch
to a virtual console to run
the tests. To do this, press ctrl+alt+f3
to switch to a virtual console (and log in), and
either ctrl+alt+f1
or ctrl+alt+f2
to switch back to your graphical session.
You might also want to install cargo-update to easily keep your tools up to date, though it is in no way required.
Configuring your editor
These steps are not required, and most of this isn't specific to Burn, but it's definitely helpful if you haven't already done it.
VSCode
Install the following extensions:
- rust-lang.rust-analyzer for Rust syntax and semantic analysis
- tamasfe.even-better-toml for TOML syntax and semantic analysis
- serayuzgur.crates for managing dependencies
- vadimcn.vscode-lldb for debugging
Setting up the Debugger
To use the debugger, follow these steps:
- Open
Command Palette
withCtrl+Shift+P
orF1
and typeLLDB: Generate Launch Configurations from Cargo.toml
then select it, this will generate a file that should be saved as.vscode/launch.json
. - Select the configuration from the "run and debug" side panel, then select the target from the list.
Since this repo has
debug = 0
in the rootCargo.toml
to speed up compilation, you need replace it withdebug = true
in the rootCargo.toml
when using a debugger and breakpoints withlaunch.json
settings. - Now you can enable breakpoints on code through IDE then start debugging the library/binary you want, like in the following example:
If you're creating a new library or binary, keep in mind to repeat step 1 to always keep a fresh list of targets.
Have another editor? Open a PR!
Testing
Test for Tensor Operations
Test for tensor operations (generally of the form: given this input, expect it match or approximate
this output) are defined only in
crates/burn-tensor/src/test/ops
and not in the backends (with the exception of burn-autodiff
). The tensor operation tests are
added to the testgen_all
macro rule in
crates/burn-tensor/src/tests/mod.rs
.
This is then propagated to the existing backends without any additional work.
Test for Autodiff
Tests for autodiff go under burn-autodiff/src/tests and should verify backward pass correctness. For binary tensor operations, both the left and right sides need to be verified.
Here's an easy way to define tests for a new operation's backward pass:
- Use small tensors with simple values.
- Pop open a terminal, launch
ipython
and importnumpy
then do the calculations by hand. You can also use Google Colab so you don't have to install the packages on your system. - Compare the actual outputs to the expected output for left-hand side, right-hand side.
For float tensors, it is advised to use
actual_output_tensor.into_data().assert_approx_eq(&expected_tensor_data,3)
instead of
assert_eq!(...
due to occasional hiccups with floating point calculations.
Project Architecture
This section documents most major architectural decisions with the reasoning behind them.
Sections
Module
Modules are a way of creating neural network structures that can be easily optimized, saved, and loaded with little to no boilerplate. Unlike other frameworks, a module does not force the declaration of the forward pass, leaving it up to the implementer to decide how it should be defined.
Additionally, most modules are created using a (de)serializable configuration, which defines the structure of the module and its hyperparameters. Parameters and hyperparameters are not serialized into the same file, and both are normally necessary to load a module for inference.
Optimization
Optimization is normally done with variants of gradient descent, and it is important to provide an easy API for optimizing modules.
Constraints
- Users should be able to control what is optimized. Modules can contain anything for maximum flexibility, but not everything needs to be optimized.
- Optimizers should have a serializable state that is updated during training. Many optimizers keep track of previous gradients to implement some form of momentum. However, the state can be anything, not just tensors, allowing for easy implementation of any kind of optimizer.
- The learning rate can be updated during training. Learning rate schedulers are often used during training and should be considered as a key aspect.
Solution
In the following, the Module
trait is defined in
crates/burn-core/src/module/base.rs
and the Optimizer
trait is defined in
crates/burn-core/src/optim/base.rs
The solution to this problem comprises multiple parts. Firstly, the Optimizer
trait is quite
similar to the Module
trait, in terms of saving and loading the state. Please refer to the
serialization section for more details.
Secondly, two traits were created. The Optimizer
trait is general and relatively unopinionated,
with a simple step
method that takes a learning rate, a module, and the gradients. The other
trait, SimpleOptimizer
, aims to provide an easier API for implementing new optimizers. The goal is
to allow implementations to avoid handling missing gradients, loading and exporting records,
navigating the module parameter structure, handling tracked and untracked tensors, and other such
tasks.
Thirdly, each tensor that will be optimized needs to be wrapped into a Param
struct, which gives
them an ID used for (de)serialization and to associate the state of the optimizer to each parameter.
The Module
trait has two ways to navigate over parameters. The first one is the map
function,
which returns Self
and makes it easy to implement any transformation and mutate all parameters.
The second one is the visit
function, which has a similar signature but does not mutate the
parameter tensors.
SimpleOptimizer
Located in
crates/burn-core/src/optim/simple/base.rs
,
the SimpleOptimizer
has two major assumptions:
- The state of the optimizer is linked to each parameter. In other words, each parameter has its own optimizer state, decoupled from the other parameters.
- The state of the optimizer implements
Record
,Clone
, and has a'static
lifetime.
The benefits of those assumptions materialize in simplicity with little loss in flexibility. The state associative type is also generic over the dimension, making it extremely easy to include tensors in the state that share the same dimensionality as its parameter.
To wrap a simple optimizer into the more general Optimizer
trait, the OptimizerAdaptor
struct is
used.
OptimizerAdaptor
Located in in
crates/burn-core/src/optim/simple/adaptor.rs
,
the OptimizerAdaptor
is a simple struct composed of a SimpleOptimizer
and a hashmap with all
records associated with each parameter ID.
When performing an optimization step, the adaptor handles the following:
- Updates each parameter tensor in the given module using the
Module::map
function. - Checks if a gradient for the current tensor exists.
- Makes sure that the gradient, the tensor, and the optimizer state associated with the current parameter are on the same device. The device can be different if the state is loaded from disk to restart training.
- Performs the simple optimizer step using the inner tensor since the operations done by the optimizer should not be tracked in the autodiff graph.
- Updates the state for the current parameter and returns the updated tensor, making sure it's properly registered into the autodiff graph if gradients are marked as required.
Note that a parameter can still be updated by another process, as it is the case with running
metrics used in batch norm. These tensors are still wrapped using the Param
struct so that they
are included in the module's state and given a proper parameter ID, but they are not registered in
the autodiff graph.
Serialization
An important aspect of a deep learning framework is the ability to save and load models from disk. Despite appearing as a simple feature, it involves numerous constraints that require a proper solution.
Constraints
-
Users should be able to declare the precision of the model to be saved, independent of the backend in use.
The modules should not be duplicated in RAM in another precision to support this. Conversion should be done lazily during (de)serialization.
-
Users should be able to add any field to a module, even fields that are not serializable.
This can include constants, database connections, other module references, or any other information. Only parameters should be serialized since the structure of the module itself should be encapsulated with module configurations (hyperparameters).
-
Users should be able to declare the format in which the module should be saved.
This can involve saving to a compressed JSON file or directly to bytes in memory for
no-std
environments. -
Users should be able to create a module with its saved parameters without having to initialize the module first.
This will avoid unnecessary module initialization and tensor loading, resulting in reduced cold start when dealing with inference.
In addition to all of these constraints, the solution should be easy to use.
Solution
In order to be able to add any field to a module without requiring it to be (de)serializable, we decouple the module type from its state. We create a new type for each module that only contains the parameters that need to be saved. To generate that type automatically, the user must either declare which field is a parameter or a constant, or we assume that each field implements the module trait.
The second solution was chosen as it simplifies the code generation and reduces the size of the user
API. This means that the Module
trait should be implemented by
primitive types.
The following diagrams highlight the main types and traits used in the solution.
Module Serialization Types
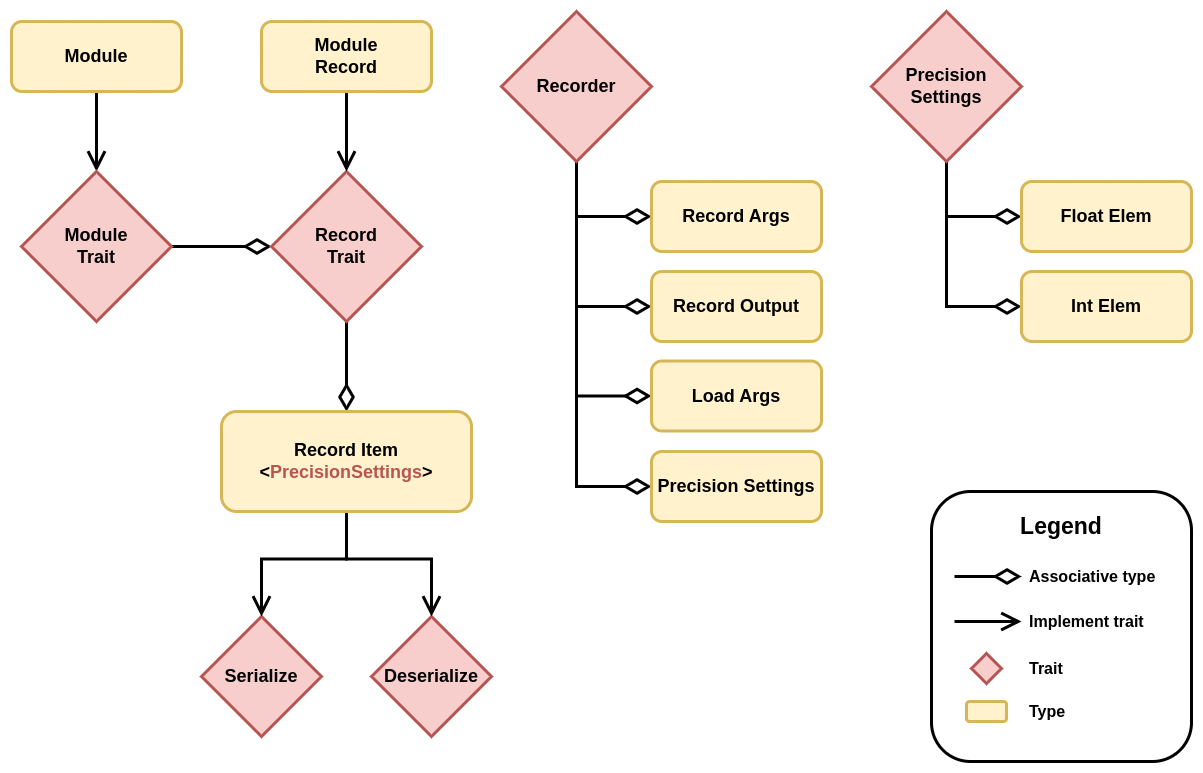
The way the types interact with each other is pretty straightforward. First, a module can be
converted into a record using into_record()
. Note that tensors can be cloned, but it won't
actually copy any data; it will simply create another reference to the same data.
Then, a Recorder
instance can be used to serialize any record. The Recorder
has the
PrecisionSettings
type as associate type, so any record will be serialized using the settings
provided at the creation of the Recorder
instance. Note that tensors implement record, and their
item is just a wrapper struct that contains information about the precision in which the tensor
should be saved or loaded. No actual copy of the tensor is made until this point. The tensor is
converted to the TensorData
struct and then converted into the specified precision only when
serialize()
or deserialize()
are called, which makes the whole process lazy.
To recapitulate, the Module
trait has an associated type that implements Record
, which only
contains the parameters of the model. The Record
trait has a generic associated type (GAT) that
specifies a family of types that can be (de)serialized given any PrecisionSettings
. Records are
therefore decoupled from the backend in use, and the saved items can be loaded on any backend with
any precision, since the conversion is type-safe and done when serialize()
and deserialize()
are
called. All of the types are generated using simple derive macros without any conditional statements
or complex syntax, as Record
and Module
are implemented for all primitive types. This makes the
code simple and easy to maintain. In addition, you can extend the current system with your own
Recorder
and PrecisionSettings
to control how your modules should be saved and loaded.
Pros
- All constraints are respected.
- The code is simple and easy to maintain, with very few conditional statements. It is just recursive data structures, where all the complexity is handled by the framework in primitive implementations.
- The user API is simple and small, with only two derives (
Record
andModule
) and no additional attributes. - Users can create their own
Module
andRecord
primitive types, which gives them the flexibility to control how their data is serialized without having to fork the framework.
Cons
- There are more types, but most of them are automatically generated and single-purpose, so users don't need to interact with them for common use cases. However, they can do so if necessary.
- When instantiating a new record manually, each field must be set to something, even if the type
itself is
()
, which represents no value. Since the code generation step uses associative types, it doesn't know that a field type is actually nothing. Creating a record manually without using the generated functioninto_record
or loading it from a file is only useful to load a set of parameters into a module from an arbitrary source. Using the record may not be the optimal solution to this problem, and another API could be created in the future.
Compatibility
Record may become incompatible with previous versions of Burn, depending on the chosen format. The more compact format (bincode) store minimal information about the type, making it significantly smaller but less resilient to type changes such adding an optional field. At some point, it might be necessary to provide a translation script that can translate a more resilient format from a previous version to a more compact one.
Tensor
A proper deep learning framework should have a fast tensor implementation with autodiff support, and Burn is no exception. The tensor API abstracts away backend implementation details and focuses on usability without compromising performance. To make it as easy as possible to use, there is only one tensor type, which is different from multiple tensor and deep learning crates in Rust. Generic parameters are used instead to specialize the tensor type.
- B: Backend: The first argument is the backend on which the tensor implementation lies.
- const D: usize: The second argument is the dimensionality of the tensor.
- K: TensorKind: The third argument is the tensor kind, which can be either Float, Int or Bool. By default, the tensor kind is set to Float, so for most tensors, the kind argument is not necessary.
Having one struct for tensors reduces the complexity of the tensor API, which also means less duplicated documentation to write and maintain.
Tensors are thread-safe, which means that you can send a tensor to another thread, and everything will work, including auto-differentiation. Note that there are no explicit in-place tensor operations since all tensor operations take owned tensors as parameters, which make it possible to mutate them. Tensors can be shared simply by cloning them, but if there is only one reference to a tensor, the backend implementation is free to reuse the tensor's allocated data. For more information about how it is done, you can have a look at this blog post.
Tensor Operations
Operations on Tensors (sometimes shortened to Ops) are defined in traits (generally part of the Backend Supertrait) and implemented for the Tensor struct. The appropriate parent trait of an operation depends on the type of operation:
base
=> All tensor kinds should implement these operations (reshape, into_data, etc.). The implementation is in crates/burn-tensor/src/tensor/api/base.rs.numeric
=> All tensors that are numeric by nature should implement these operations (Add, Sub, Div, etc.). The implementation is in crates/burn-tensor/src/tensor/api/numeric.rs.Float
=> Tensor operations are only available for float tensors. The implementation is in burn-tensor/src/tensor/api/float.rs.Int
=> Tensor operations are only available for int tensors. The implementation is in burn-tensor/src/tensor/api/int.rs.bool
=> Tensor operations are only available for bool tensors. The implementation is in burn-tensor/src/tensor/api/bool.rs.
Numeric
is directly implemented for Float
and Int
tensors, and in general, The implementations
for these methods are calling the corresponding {Int|Float}
method defined in the backend
supertrait.
Anything that is implemented by numeric should have an implementation in the {Int|Float}
traits,
though it may be avoidable if the operation for one type requires casting to the other type. To
provide an example, powf
should be implemented for Int
tensors, but it should not be an Int
Tensor Operation. The LHS should be converted to a float, and the output should be converted back to
an int. So it's possible to avoid implementing IntTensorOp
altogether.
Additionally there are some operations that should be defined as functions instead of tensor op methods. These are:
module
=> These should be exported as functions instead of methods on tensors. The implementation
is in
crates/burn-tensor/src/tensor/ops/module.rs.
activation
=> These should also be exported as functions instead of methods on tensors. The
implementation is in
crates/burn-tensor/src/tensor/ops/activation.rs.
Note that some activations are just a combination of backend operations and are not declared in
there.
Backend
The Backend trait abstracts multiple things:
- Device type
- Float tensor type
- Bool tensor type
- Int tensor type
- Float element type
- Int element type
- Float tensor operations (kernels)
- Int tensor operations (kernels)
- Bool tensor operations (kernels)
Element types
Warning: there are plans to change this architecture in the near future.
Even though having one type for tensors is convenient for the tensor API, it can be cumbersome when implementing a backend. Therefore, backends can decide, through associated types, what types they want to use for their int, float, and bool tensors. Since float and int can have multiple precisions, the float and int element types are also associated types that must be declared by the backend.
Note that the backend chooses the precision and not the user. Since not all backends will support
the same element types, no assumptions must be made. Therefore, there are no methods on tensors to
change the precision, except for the to_full_precision
function, which ensures numerical stability
on the current backend. Backend implementations can provide a way to choose the precision, which can
be accomplished with a generic parameter (e.g. NdArray<f32>
).
Operations
To be as general as possible, tensor operations are implemented as plain functions. There is no object or self, just functions that take tensors as input and often return tensors as output as well. Backend implementations are free to use their own patterns to implement these kernels. Note that Burn is a dynamic graph deep learning framework, so backends may have to implement asynchronous kernel executions for performance reasons.
Autodiff
As of now, there is only one backend decorator that supports autodiff. It follows the decorator
pattern, making any backend differentiable. However, the AutodiffBackend
trait abstracts how
gradients are calculated, and other approaches to autodiff might be added later. For more
information about how the current autodiff backend works, you can read this (slightly outdated)
blog post.
Guides for Contributors
The following guides are meant to help contributors accomplish specific tasks, such as adding new operations to Burn or generating test models for burn-import
.
ONNX to Burn Conversion Tool: Development Guide
This guide offers in-depth design insights and step-by-step procedures for developers working on the ONNX to Burn conversion tool. This tool allows the importation of ONNX models into the Burn deep learning framework written in Rust. It converts both ONNX models to Rust source code and model weights to Burn state files.
For an introduction to ONNX import in Burn, see this section of the Burn book.
Table of Contents
- ONNX to Burn Conversion Tool: Development Guide
Design Overview
Design Goals
- Perform best-effort conversion of ONNX models to Rust source code via Burn APIs.
- Convert ONNX model weights to Burn state files.
- Support ONNX models generated by PyTorch (ONNX Opset 16).
- Produce easy-to-understand and modifiable models.
- Ensure the generated models are trainable using Burn APIs.
Design Decisions
- Limit interaction with ONNX to the Intermediate Representation (IR) stage to simplify the process.
- Ensure operator behavior consistency across different OpSet versions.
- Exclude any ONNX/Protobuf-specific logic from the Burn graph.
The conversion process involves three main stages:
- Convert ONNX model to Intermediate Representation (IR).
- Translate IR to a Burn graph.
- Generate Rust source code from the Burn graph.
Adding New Operators
To extend burn-import
with support for new ONNX operators, follow these steps:
-
Create PyTorch Script: Place a PyTorch script using the new operator under
crates/burn-import/onnx-tests/tests/<op>/<op>.py
. Make sure to print both input and output tensors for end-to-end testing. -
Generate ONNX Model: Run the PyTorch script to produce an ONNX model.
-
Visualize ONNX Model: Use Netron to verify the ONNX model contains the expected operators.
-
Generate IR and Burn Graph: Navigate to crates/burn-import/ and run:
cargo r -- ./onnx-tests/tests/<op>/<op>.onnx ./out
-
Implement Missing Operators: If you encounter an error stating that an operator is unsupported, implement it. The
./out/my-model.graph.txt
should provide relevant information. -
Inspect Generated Files: The
my-model.graph.txt
contains IR details,my-model.rs
holds the Burn model in Rust code, andmy-model.json
includes the model data. -
Add End-to-End Test: Include the test in crates/burn-import/onnx-tests/tests/onnx_tests.rs. Further details can be found in the onnx-tests README.
Implementing a New Operator
To extend the capabilities of the Burn library by supporting new operations imported from ONNX
graphs, developers must go through a few systematic steps. Here, we detail the process, using the
implementation of the Squeeze
operation to illustrate points as needed. All file/directory paths
are relative to burn/crates/burn-import/
.
Step 1: Visibility
To make a new operation accessible to the rest of the Burn project, you need to declare the module
within the
mod.rs
file
located in the src/burn/node/
directory.
Step 2: Node Implementation
Within Onnx-IR
If the node type does not exist within the
NodeType
enum,
it will need to be added (support for custom operators is planned). If the node might be provided an
input which is a constant or the output of an identity node, it will need to be added to the list of
nodeTypes
checked for constants.
The node will need to be added to dim_inference
, and in most cases the work parsing side will be
done. If a node requires extra parsing (such as handling an edge case like potentially remapping an
unsqueeze to a reshape) the best place for that is after check constants and prior to dim_inference
in
OnnxGraphBuilder::Build
Within burn-import
Create a new file named <operation_name>.rs
in the src/burn/node/
directory.
This file will define the structure and functionality of your new operation. By convention, the
necessary information for carrying out an operation is encapsulated within a struct named
<operation>Node
. For the Squeeze
operation, we defined a
struct called SqueezeNode
that holds necessary information about the input tensor, output tensor, and axes for the operation.
If implementing a unary or binary operation, please see note below.
The core of integrating a new operation involves implementing the NodeCodegen
trait for your node.
This trait defines how the node generates code during the graph compilation process. The
implementation must provide methods to define input and output types, to generate the forward pass
code, and to encapsulate the node into the more general Node
structure. Specifically:
output_types
andinput_types
return the tensor (or element) types for the output and inputs of the node, respectively.forward
generates the Rust code that performs the operation during the execution phase. Thequote!
macro is used to generate rust code. Ensure that this is syntactically correct using Burn code.into_node
wraps the specific node in a generalNode
type, facilitating its inclusion in the broader Burn graph structure.
This file is also where you would put test_codegen_nodes()
, to make sure that the generated code
works within the Burn library.
For unary and binary operations: The implementation of NodeCodegen
is mostly implemented in
binary.rs
and
unary.rs
,
so each new operation only has to define a method to execute the function on the input(s) token
stream.
Step 3: Registering New Operations
Register the NodeType::<operation>
and
create an <operation>_conversion(node: Node)
function,
both in src/onnx/to_burn.rs
.
Registering new operations in the ONNX -> Burn Conversion
To integrate new operations from an ONNX graph into the Burn framework, each operation must be
registered within the ONNX graph conversion process. This is done in the src/onnx/to_burn.rs
file,
where the conversion from ONNX nodes to Burn nodes is orchestrated.
In the into_burn()
method of the OnnxGraph
struct, operations are matched with their
corresponding conversion functions. This method iterates over each node in the ONNX graph and,
depending on the node type, calls a specific conversion function that translates the ONNX node into
a corresponding Burn node.
#![allow(unused)] fn main() { impl OnnxGraph { pub fn into_burn<PS: PrecisionSettings + 'static>(self) -> BurnGraph<PS> { let mut graph = BurnGraph::<PS>::default(); let mut unsupported_ops = vec![]; for node in self.nodes { match node.node_type { NodeType::Add => graph.register(Self::add_conversion(node)), // Other operations... NodeType::Squeeze => graph.register(Self::squeeze_conversion(node)), // Add new operations here } } } } }
Here, the NodeType::Squeeze
matches the ONNX node type with the squeeze_conversion()
function
that you define to handle the specific attributes and settings of a Squeeze operation.
Define the Conversion Function
Each operation conversion function extracts necessary information from the ONNX node and constructs
a corresponding Burn node. The structure of these functions generally includes:
- Extracting input and output tensors from the node.
- Retrieving and processing operation-specific configurations.
- Calling
<operation>_config()
to parse ONNX node configurations. - Creating an instance of the appropriate Burn node (defined in step 2) using this information.
Step 4: Create a Config Function
Create an <operation>_config(curr: &Node)
in src/onnx/op_configuration.rs
.
The squeeze_conversion()
function in src/onnx/to_burn.rs
from the previous step calls the
squeeze_config()
function in src/onnx/op_configuration.rs
in order the parse the ONNX node's
attributes to extract parameters specific to the Squeeze operation. In this case, the axes along
which the squeeze operation is performed.
π Info: Understanding Generic
config
PatternsThe
<op>_config()
functions follow a similar pattern:
- Extract tensor or scalar types for inputs and outputs.
- Validate the input structure and types for each node, ensuring they conform to expected formats (panicking if not).
- Parse and convert configurations or parameters specific to each operation.
- Create and return a node specific to the operation, initialized with extracted values and configurations.
For example, config functions handle specific settings like kernel size for pooling or handling different tensor and scalar types for power operations.
These functions translate the more varied and flexible structure of ONNX nodes into the more structured and type-safe environment of Rust and the Burn framework. Spec compliance is dealt with here.
Step 5: Dimension Inference
If needed,
create a dimension inference function,
called <operation>_update_output(node: &mut Node)
in src/onnx/dim_inference.rs
. If dimensions
remain unchanged, use the same_as_input()
function, for example
NodeType::AveragePool1d => same_as_input(node)
. Match the NodeType
to the function in the
dim_inference()
match block.
Dimension inference is an important step in the conversion process where Burn determines the
dimensions of each output tensor based on the operation.
The dim_inference()
function is responsible for determining the dimensions of the output tensors for each node in the
graph. It does this by:
- Matching the Node Type: The function uses a
match
statement on thenode_type
of each node to apply the correct dimension inference logic depending on the operation. - Applying Operation Specific Logic: For each operation, a specific inference function is called that encapsulates the rules for how output dimensions should be derived from the inputs.
For the Squeeze operation, the dimension inference is handled by the squeeze_update_output()
function, which is specifically tailored to handle the nuances of the squeeze operation, which is
currently not that nuanced. The output tensor should be (dimensions of input tensor) - 1.
π Info: How
squeeze_update_output()
Works
- Validation of axes input: We first check if the second input of the node contains a list of integers, which represent the axes along which the squeeze operation is applied. The function also validates that only one axis is specified for squeezing, ensuring that the operation's requirements within Burn are followed.
- Extracting input dimensions: The input tensor's dimension is extracted from the first input.
- Configuring output dimensions: The output tensor's dimensions are then set to be one less than the input tensorβs dimensions, reflecting the reduction in dimensions caused by the squeeze operation.
- The function includes several checks that throw errors (panics) if the inputs do not meet the expected types or configurations, such as when the axes are not provided as an integer list or if the input type is not a tensor.
By invoking this function within the dim_inference()
match block, the output dimensions of each
node are updated before the graph is finalized. This ensures that all subsequent operations within
the graph can rely on correct tensor sizes, which is critical for both compiling the graph and for
runtime execution efficiency.
If something is amiss (ie weird panics are happening), after doing this step and the dimensions of your output tensor differs from the dimensions of your input, see the warning at the very end.
Step 6: Integrate into the Graph Building Process
When a new node type is introduced, it must be added to the
Node<PS: PrecisionSettings>
enum
and
match_all!
macro
in src/burn/node/base.rs
.
The Node
enum abstracts over different types of operations (nodes) within a network graph. Each
variant of the enum corresponds to a specific type of operation, and it encapsulates the
operation-specific data structures (like SqueezeNode1
) that was
defined in step 2.
Step 7: Add Newly Supported Op!
As a reward, add an extra check to SUPPORTED-ONNX-OPS.md!
Misc:
π§ Warning: Dimension Changes
If your operation changes the dimensions of the input tensor, you may need to modify the
LIFT_CONSTANTS_FOR_NODE_TYPES
enum insrc/onnx/from_onnx.rs
by adding theNodeType
of your operation to it.
Testing
- Unit tests for the Burn graph to Rust source code conversion are mandatory.
- End-to-end tests should include a test ONNX model and its expected output for each operator.
Resources
Adding a New Operation to burn
Let's discuss how one might go about adding new operators to Burn, using the example of the pow operator added in this PR.
Adding the Op to burn-tensor
burn-tensor
is the crate that defines all tensor operations that need to be implemented by the
various backends. The core of this lies in
crates/burn-tensor/src/tensor/api/numeric.rs,
which is home to the numeric trait and its implementation for the different tensor types. The
numeric trait is the home of all tensor operations that are numeric in nature and that are shared by
Int
and Float
Tensor types. More information on the relationship between Tensor modules can be
found under the section for Tensor Architecture.
Here is where pow was added to crates/burn-tensor/src/tensor/api/numeric.rs
:
- for the
Tensor<Backend, Dimension, Kind>
struct - for the numeric trait
- for the implementation of numeric for float and int
Tensor is a struct that has a single member: primitive
(defined
here),
that is defined by its
Kind
:
one of Bool
, Float
, or Int
(those linked in 3). These call the ops for that data type defined
in the
Backend
supertrait1. This is the trait that is then implemented by the different burn-
backends (such as burn-ndarray
and burn-wgpu
) which must implement the functions if no default
is provided.
In this case, we don't need to worry about Bool
Tensors. Ops for Float
is implemented under
crates/burn-tensor/src/tensor/ops/tensor.rs
,
and for Int
under
crates/burn-tensor/src/tensor/ops/int_tensor.rs
.
The current convention is ops of each type, if not unique to that type, are prefixed with the type.
So powf
and sundry would be defined as int_powf
for IntTensorOps
and float_powf
for
FloatTensorOps
. If an op is unique to a type, then it should be implemented under
burn-tensor/src/api/{type}.rs
. For example, here is an implementation for
sin
under crates/burn-tensor/src/api/float.rs
which obviously doesn't make sense for Int
or Bool
tensors.
The Int
Tensor function uses the ones defined for Float with 2 extra casts (LHS to a Float
tensor, Output to an Int
). Given that the rest of the code will only look at the float
implementations.
Adding Tests
Additional Tests should be added to burn-tensor
under
crates/burn-tensor/src/tests/ops/{op_name}.rs
,
inserting the module name into crates/burn-tensor/src/tests/ops/mod.rs
. Then add it to the
testgen_all
macro under crates/burn-tensor/src/tests/mod.rs
. This macro is called from the
lib.rs
file in each backend, which autogenerates the tests for that specific backend. It isn't
necessary to define tests in the backends directly, save for those that require specific testing
such as burn-autodiff
.
Adding the Op to burn-autodiff
Since this is probably the hardest and the least straightforward, we'll cover this backend
separately. burn-autodiff
enables other backends to use autodifferentiation2. Ops for
float types are implemented in
crates/burn-autodiff/src/ops/tensor.rs
and need to:
- Define a unit struct 3 that implements a backward (pass) function
- Within the backward function, as this is an elementwise binary operation it implements the binary
function (from
backward.rs
under the same directory), the last 2 arguments are two closures that define the left and right partial derivatives. - Then define what happens when a specific operation is tracked or untracked, where untracked just calls the function in the normal way, and tracked sets the execution the backward function defined above.
- When tracked, operations are part of the autodiff graph and must save the needed information to efficiently perform their backward pass later. If the information is light (such as a shape), it should be directly saved in the state. If the operation's inputs are needed to compute the backward pass, it should be checkpointed rather than saved. This will allow the input to be provided lazily at the backward pass depending on the checkpointing strategy.
- An operation must also be identified as compute-bound (
.computeBound()
) or memory-bound (.memoryBound()
) for gradient checkpointing. Compute-bound operation are heavy to compute (for instance matmul or convolution), which means that even with checkpointing they will save their output for the backward pass and not recompute it. Memory-bound operations are more trivial (likepowf
which only performs one small operation per tensor entry), so it can be beneficial to recompute them during the backward pass instead of saving their whole forward output to memory. Operations registered as memory-bound need to know their parents (.parents()
method) and how to recompute their forward pass during the backward pass (with a struct that implementsRetroForward
), using their parents' outputs.
The above steps are mostly boilerplate, so you can often just copy the contents of another similar op, change the name of the structs, and ensure that either both sides have the data they need (if they need to have a copy of the opposite sided tensor, clone its contents).
Computing derivatives
For those that need it, here is a quick refresher on the necessary calculus. If you are familiar with how to calculate partial derivatives, you can skip this section.
Since pow
is a binary operation, the left and right functions are the partial derivatives with
respect to the left and right sided tensors.
Let's define the operator as a function \(f(x,y)=x^{y}\) , where \(x\) is the left hand tensor and \(y\) is the right handed tensor. The two closures are defining the partial derivatives of \(f\) with respect to \(x\),\(y\). Treat the other variables as a constant
$$\frac{\delta }{\delta x} (x^{y})= y \cdot x^{y-1}$$ is the left handed closure, and
$$\frac{\delta }{\delta y} (x^{y}) = x^{y} \cdot ln(x)$$
is the right. If you aren't sure how to calculate these by hand, it is recommended to use symbolab, plug in your operator in terms of \(x\) and \(y\), and just swap out the variable \(x\)|\(y\) in the partial derivative to get the other side.
Testing autodiff
For testing the autodiff
operations, please refer to
this section.
Adding the Op to other backends
Most of these are fairly straightforward implementations. For reference here's pow's float implementation for torch, ndarray and candle backends:
- Torch implementation in crates/burn-tch/src/ops/tensor.rs and the Op used in crates/burn-tch/src/ops/base.rs
- NdArray in crates/burn-ndarray/src/ops/tensor.rs
- Candle in crates/burn-candle/src/ops/tensor.rs
This is where any calculation happens currently. Playing a guessing game with method names and seeing what completions are suggested will take you far. If you are having trouble figuring out how to do it from the docs for that backend, try searching github for relevant function calls.
Adding the Op to fusion, JIT and cubecl backends
Adding an operator to these backends can be fairly straightforward, though due to what these backends are for, involves a bit more indirection. Fusion and jit, like autodiff, are not target backends as much as backends that enable certain functionality for other backends, in this case kernel fusion or just-in-time compilation. Adding the operator won't involve doing any calculation, you'll just be describing how the generated code should look. Most of this can be copy/pasted/adjusted from other functions.
Here's how powf was added to burn-fusion
:
- Added powf to the float ops under
crates/burn-fusion/src/ops/float.rs
- Added powf to the
NumericOperationDescription
enum under crates/burn-fusion/src/stream/operation.rs - Added powf to the implementations of
NumericOperationDescription
enum under crates/burn-fusion/src/stream/context.rs
The way cubecl
handles tensor-scalar operations is by transforming both into a sequence of
vectorized scalar operations. Since powf already existed in cubecl
, it was pretty easy to reuse
the existing implementation for the situation where both sides of the operation were tensors. The
cubecl
crate is primarily concerned with how the operation is compiled and executed by the gpu.
The actual implementation is defined in burn-jit
.
Here is where code was added for powf in burn-jit
and cubecl
:
- to the implementation of
FloatTensorOps
undercrates/burn-jit/src/ops/float_ops.rs
- the function being called was added to crates/burn-jit/src/ops/numeric.rs
- the operator was defined in
cubecl-core/src/ir/operation.rs
- how the operation looks to the gpu was added to
crates/burn-jit/src/fusion/on_write/ir.rs
- the mappings between the gpu operation and the CPP, WGSL and SPIR-V instructions were added to
cubecl-cpp/src/shared/base.rs
,cubecl-wgpu/src/compiler/wgsl/compiler.rs
andcubecl-spirv/src/instruction.rs
- the instructions themselves were added for WGSL to
instruction op enum in
cubecl-wgpu/src/compiler/wgsl/instructions.rs
, and the actual instruction in wgsl here, for CPP in the enum herecubecl-cpp/src/shared/instruction.rs
and the actual instruction herecubecl-cpp/src/shared/binary.rs
We needed to generate some custom WGSL code for powf in WGSL, primarily due to issues with proper
case handling of the wgsl pow function, like 0 to the 0 power being 1, and any negative number to an
even power being positive. We reused as much as the existing logic as possible, and then branched at
the last point based off the var type of the rhs.
See here.
For most operations, you shouldn't need to add to cubecl-wgpu/src/compiler/wgsl/extension.rs
unless the operation isn't native to WGSL.
For functions that need a complex kernel without a direct mapping to a base instruction, simply use
the cube
macro (see
the cubecl
book).
Adding the Op to burn-import
Generating the ONNX test files or tests is already covered in the ONNX to burn guide; this is more about the specific changes you need to make when adding new operators after you have generated the tests.
Changes will need to be made to both onnx-ir
and burn-import
. The code within onnx-ir
defines
how to parse the nodes in an onnx file and produces the intermediate representation. The code within
burn-import
is divided into two sections: src/onnx
and src/burn
. The code under the former
maps that intermediate representation to one used for code generation and the latter defines how to
generate code for the operator you've implemented earlier in this guide.
So when you are loading a model, the operator is first parsed to an intermediate representation
defined by burn-import
and then mapped to a Burn operation defined under src/burn/node
; the
mapping from onnx to burn is aptly defined in src/onnx/to_burn
Let's review the changes made for powf starting from src/burn
and moving to src/onnx
:
- Determine the type of operator and add your operator to the appropriate node (operation) type, in
this case
BinaryNode under
crates/burn-import/src/burn/node/binary.rs
along with itsto_str
definition - Add an arm to the match statement inside the
into_burn
function in crates/burn-import/src/onnx/to_burn.rs for the ONNXNodeType
(which corresponds to an op in the ONNX spec), and make an{op}_conversion
function that maps the ONNX node to the binary type - Specify how dimensions for the output should be derived in crates/onnx-ir/src/dim_inference.rs
And you're done! Congrats, you just fully added a new operation to burn, and we are all one step closer to the answer to Are we learning yet? being "Yes, and it's freaking fast!". Buy yourself a coffee.
for more on supertraits see the advanced trait section of the rust book
wiki link for automatic differentiation
for more information on unit structs see the defining and instantiating structs section of the rust book
Frequently Encountered Issues
This is a collection of issues people have encountered and asked about on the Discord server. This section is separated from the guides since it can involve lots of details that are only relevant to a small subset of contributors.
Issues encountered while adding ops
Below are some of the issues that were encountered while adding ops to the project. If you encounter an issue while adding an op that isn't listed here, and it's not obvious how to fix it, you can add it to this list or reach out on the Discord server if you need help.
Off by .000001 errors
---- fusion::base::tests::maxmin::tests::test_mean_dim_2d stdout ---- thread 'fusion::base::tests::maxmin::tests::test_mean_dim_2d' panicked at burn-wgpu/src/fusion/base.rs:185:5: assertion `left == right` failed left: Data { value: [1.0, 4.0], shape: Shape { dims: [2, 1] } } right: Data { value: [0.99999994, 3.9999998], shape: Shape { dims: [2, 1] } } ----
tests::maxmin::tests::test_mean_dim_2d stdout ---- thread 'tests::maxmin::tests::test_mean_dim_2d' panicked at burn-wgpu/src/lib.rs:49:5: assertion `left == right` failed left: Data { value: [1.0, 4.0], shape: Shape { dims: [2, 1] } } right: Data { value: [0.99999994, 3.9999998], shape: Shape { dims: [2, 1] } }
If you encounter this, swap out the assert_eq!
in the failing test for
tensor1.to_data().assert_approx_eq
with 3
as the second argument. The second arguments specifies
the level of precision: 3
is equivalent to a less than 10-3 (0.001) difference between
the elements of the two tensors.
Mismatched types and missing functions
error[E0308]: mismatched types --> {burn_dir}/target/debug/build/onnx-tests-fed12aaf3671687f/out/model/pow.rs:48:45 | 48 | let pow1_out1 = input1.clone().powf(input1); | ---- ^^^^^^ expected `f32`, found `Tensor<B, 4>` | | | arguments to this method are incorrect | = note: expected type `f32` found struct `Tensor<B, 4>`
note: method defined here --> {burn_dir}/burn-tensor/src/tensor/api/float.rs:65:12 | 65 | pub fn powf(self, value: f32) -> Self { | ^^^^
error[E0599]: no method named `powf_scalar` found for struct `Tensor` in the current scope --> {burn_dir}/target/debug/build/onnx-tests-fed12aaf3671687f/out/model/pow.rs:50:35 | 50 | let pow2_out1 = pow1_out1.powf_scalar(cast1_out1); | ^^^^^^^^^^^ method not found in `Tensor<B, 4>`
error[E0599]: no method named `powi` found for struct `Tensor` in the current scope --> {burn_dir}/target/debug/build/onnx-tests-fed12aaf3671687f/out/model/pow_int.rs:49:40 | 49 | let pow1_out1 = input1.clone().powi(input1); | ^^^^ method not found in `Tensor<B, 4, Int>` Some errors have detailed explanations: E0308, E0599.
For more information about an error, try `rustc --explain E0308`. error: could not compile `onnx-tests` (test "onnx_tests") due to 3 previous errors
If you are getting this error, you probably didn't implement your operator for the actual Tensor
struct. This issue was encountered when adding the Pow operator. The operation was added to the
FloatTensorOps
and IntTensorOp
traits, but not for the numeric trait (under
burn-tensor/src/tensor/api/numeric.rs
). This, coupled with powf
existing prior to the PR though
only for scalar values (which had been renamed, just not in the right place), led to this confusing
issue where it looked like the function was found, but the type was wrong. If that's the case, make
sure that it's implemented for the appropriate type, in this case Float
under
crates/burn-tensor/src/tensor/api/numeric.rs,
and calling the TensorOp.foo_op
defined under
crates/burn-tensor/src/ops/tensor.rs